Detail

- This event has passed.
TrAC Seminar Series – Jian-Xun Wang
November 6, 2024 @ 11:00 am – 12:00 pm
Date: November 6, 2024
Time: 11:00 AM – 12:00 PM CST
Location: 2019 Morrill Hall or https://iastate.zoom.us/j/95263587232?pwd=K1BVcURvWExFb3pUT000NlRwdFZsZz09
Title: Deep Learning Meets Numerical PDEs: A Neural Differentiable Physics Approach for Predictive Modeling
Abstract
Predictive modeling and simulation play a critical role in understanding, predicting, and controlling complex physical processes across numerous engineering disciplines. Traditional numerical methods, grounded in first-principle physics, often struggle with systems characterized by multi-scale, multi-physics phenomena, where either the governing equations are incomplete or the computational demands are prohibitive. These challenges are particularly prominent in cases requiring real-time control, design optimization, or uncertainty quantification, where iterative model queries or repeated simulations become computationally expensive. Moreover, our incomplete understanding of the underlying physics frequently leads to inaccurate mathematical models that fail to capture intricate system behaviors. Advances in sensing technologies and the explosion of available data have spurred the development of data-driven modeling techniques, providing new opportunities to enhance predictive capabilities. While deep learning (DL) techniques have demonstrated great promise, they also face limitations such as dependence on large datasets, limited interpretability, and difficulties in generalizing to new scenarios. Thus, addressing the challenges of predictive modeling demands innovative approaches that combine the rigor of physics-based models with the adaptability of data-driven techniques.
This talk introduces neural differentiable physics—a novel framework that unifies numerical partial differential equation (PDE) solvers with state-of-the-art deep learning models to enhance the modeling of complex physical systems. Unlike existing physics-informed learning approaches, such as Physics-Informed Neural Networks (PINNs), this framework integrates numerical PDE operators directly into neural network architectures via differentiable programming. By doing so, we fuse prior knowledge of physical laws, multi-scale data, and numerical techniques with the power of deep learning, leading to more accurate, efficient, and generalizable models. This hybrid approach represents a new frontier in scientific machine learning (SciML), one that bridges the gap between classical computational physics and modern AI. By leveraging both physical principles and machine learning, neural differentiable physics framework offers a transformative paradigm for solving complex multi-physics problems, with wide-ranging implications for scientific discovery and engineering applications.
Speaker Bio
Dr. Jian-Xun Wang is Robert W. Huether Collegiate Associate Professor of Aerospace Engineering in the Department of Aerospace and Mechanical Engineering at the University of Notre Dame (https://engineering.nd.edu/faculty/jian-xun-wang/). He earned his Ph.D. in Aerospace Engineering from Virginia Tech in 2017 and worked as a Postdoctoral Scholar at UC Berkeley before joining Notre Dame in 2018. Dr. Wang has a multidisciplinary research background that spans Scientific Machine Learning, Data Assimilation, Bayesian Computing, Uncertainty Quantification, and Computational Fluid Dynamics. His research focuses on the in-depth integration of advanced AI/ML techniques with physics-based numerical models, aiming to revolutionize the field of computational science in the era of “big data”. He has led research projects sponsored by multiple agencies, including NSF, ONR, NIH, AFSOR, DARPA, Google, and others. Dr. Wang is a recipient of the 2021 NSF CAREER Award and the 2023 ONR YIP Award. He is also an elected vice chair of the US Association for Computational Mechanics (USACM) Technical Thrust Area on Data-Driven Modeling.
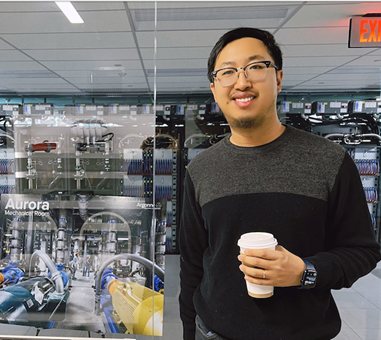